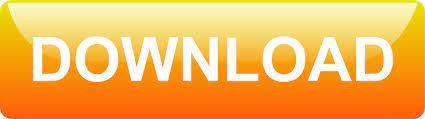

Permission is hereby granted, free of charge, to any person or company obtaining a copy of this software and associated documentation files (the "Software") from the copyright holders to use the Software for any non-commercial purpose. To do so, first create corresponding subfolders: data/newdataset/, Now that we have all data and calibrations, we need to create train, val, test splits.You can use the provided datasets as reference. The grayscale masks should be placed to data/newdataset/mask/ subfolder. We used this repository to generate the masks. Optionally, you can now generate the masks by using data/newdataset/rgb/* images as the source, to filter out, e.g., people, bicycle, cars or any other dynamic objects.This will set the data up, undistort images to data/newdataset/rgb, and calibrate the camera parameters to data/newdataset/kai_cameras_normalized.json.Run colmap_runner/run_colmap.py data/newdataset in the root folder.Copy all the images to data/newdataset/source.Create a dataset directory in data/, e.g., data/newdataset and create source and out subfolders, e.g., data/newdataset/source, data/newdataset/out.Set the path to your colmap binary in colmap_runner/run_colmap.py:13.So, if you have an image dataset, you would need to do the following: For more details, refer to the provided datasets. In addition, we also support masking via adding mask directory with monochrome masks alongside rgb directory in the dataset folder. See NeRF++ sections on data and COLMAP on how to create adapt a new dataset for training. Put the downloaded folders into data/ sub-folder in the code directory.
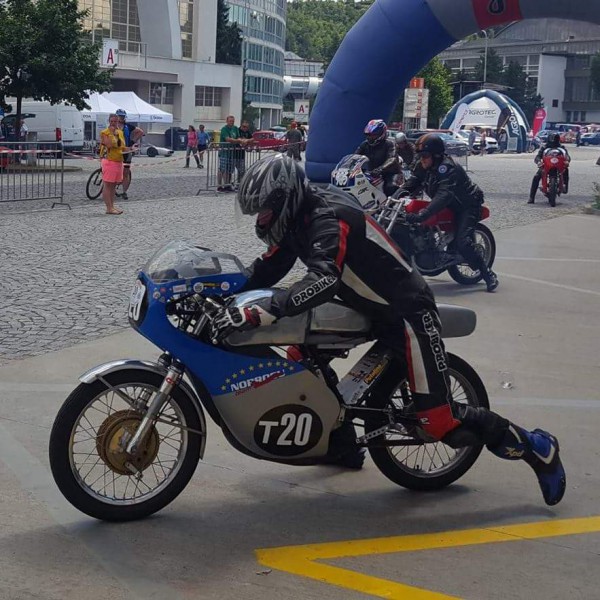
Our datasets and preprocessed Trevi dataset from PhotoTourism can be found here. Viktor Rudnev, Mohamed Elgharib, William Smith, Lingjie Liu, Vladislav Golyanik, Christian TheobaltĬodebase for ECCV 2022 paper " NeRF for Outdoor Scene Relighting".īased on NeRF++ codebase and inherits the same training data preprocessing and format.
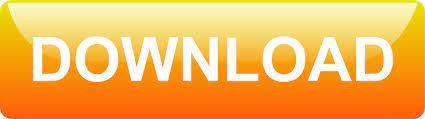